The past, present and future of machine translation.
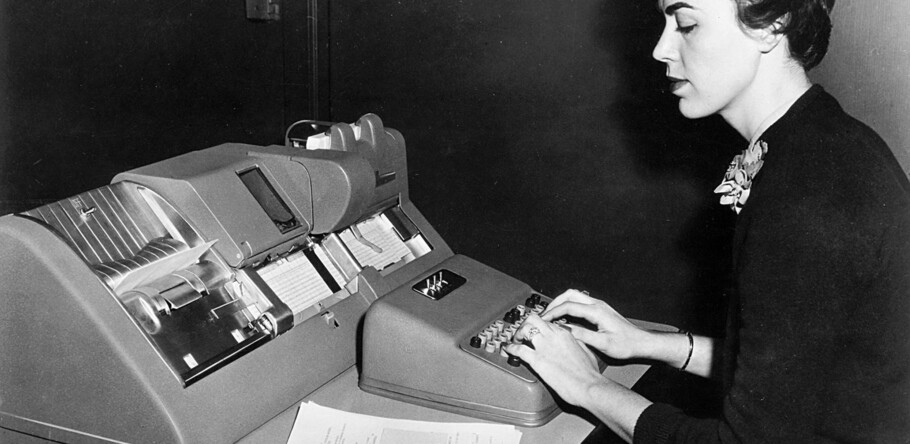
Julia Errens traces the course of machine-augmented translation, from Turing to Google Translate.
Douglas Adams had the Babel Fish, Doctor Who’s TARDIS does the job for its passengers in a process that is mostly fictional with very little science, and the unplugged rebels of the Matrix can download language skills in seconds.
Modern myths are full of machines (or ear-dwelling lifeforms) that would help humanity reclaim Babel and overcome the barriers that separate the 6,000–7,000 human languages on earth.
The scenario of automatic translation is tempting in principle and seems closer than ever, seeing as smartphones have already handed us the hitchhiker’s guide to the known galaxy by giving us handheld access to the majority of human knowledge. But before we look at where we might be headed, we should glance at where we came from, as the point of origin determines our trajectory.
On 4 March 1947, mathematician and Director of the Division of Natural Sciences at the Rockefeller Foundation in New York, Warren Weaver, sent a letter to cyberneticist colleague Norbert Wiener, reflecting on the achievements of war-time machine decoding: “One wonders whether the problem of translation might actually be a problem of cryptography.”
Like WWII’s decoding machines – developed by the likes of Alan Turing to break German military communications’ code – the earliest machine translation (MT) systems were government funded. For one, because governments felt the need during the rising tensions of the Cold War. Further, because a computer at the time would cost a considerable amount to build (not to mention taking up considerable office). There was no personal computing market, and technology was far too costly to make MT an economical pursuit for business.
Networking these campervan-sized machines globally wasn’t even on the horizon. The original struggle was to build computers that could perform rule-based translation within their own confines. The goal of this is, in essence, to teach a machine the full vocabulary and grammar of multiple languages, so that it may translate autonomously as a closed system.
In 1954, the IBM 701 was able to translate 49 sentences on chemistry from Russian into English, running one of the first non-numerical computer applications. It also took up two rooms at IBM’s New York headquarters. After the initial euphoria of the possibilities, the realities of unsound syntax and lack of grammatical correction soon caused the US government to cut back on funding. What followed were two decades of relative silence on the circuits and slow growth outside the interest of the public, until the next spike in rule-based MT crops up, and for utterly civilian purposes. In 1976, the Canadian Meteo system began translating meteorological forecasts for the French-English bilingual nation. This was more achievable than general translation, as weather forecasts work in reasonably limited stock phrases (“throughout April, scattered showers”).
As the Cold War drew to an end, it was evident that MT was shifting from military to civilian interests. This is also apparent in Jonathan Slocum’s 1985 essay, ‘Machine Translation’, which pointed out that, at the time, the Japanese were pushing the market as they considered translation “necessary to their technological survival, but have found it extremely difficult – and expensive – to accomplish by human means”. Meanwhile in the West, the biggest MT effort was the European Commission’s Eurotra, which aimed to provide translation between the nine official languages of the European Economic Community.
By the 1990s, the need for international communication was brought to the forefront of global consciousness, both by the collapse of the Soviet Union and because accelerating globalization set brands the challenge of localizing their products for foreign markets. Additionally, private consumers had been putting personal computers into their homes for nearly a decade, and were now hooking them up to the internet.
The first online MT service went live in 1992, when Compuserve started offering translations of forum content to its subscribed users from English into German.
This was followed by the 1997 launch of Altavista’s (now part of Yahoo) infamous BabelFish (named after the inner-ear dwelling lifeform from Douglas Adam’s novel The Hitchhiker’s Guide to the Galaxy that had universal simultaneous translation skills). BabelFish could translate between English, German, French, Spanish, Portuguese and Italian, and was free to use, blowing the mass market for accessible MT wide open. However, despite its novelty, BabelFish had considerable performance issues. The translated sentences were semantically challenged and unreliable when it came to picking the right translation whenever a word had multiple meanings in the goal language.
Yehoshua Bar-Hillel wrote more extensively on this issue of disambiguation in MT in his seminal 1960 essay ‘A Demonstration of the Nonfeasibility of Fully Automatic High Quality Translation’. In it, he puts forth this sentence: “Little John was looking for his toy box. Finally he found it. The box was in the pen.” He then elaborates that “no existing or imaginable program will enable an electronic computer” to come to the logical conclusion that the pen in question is a children’s playing enclosure, not a writing utensil, as that conclusion relies on knowledge that lies beyond the text, in human common sense
“What [the suggestion of MT] amounts to… is the requirement that a translation machine should not only be supplied with a dictionary but also with a universal encyclopedia. This is surely utterly chimerical and hardly deserves any further discussion.”
Well. We’ll forgive Mr Bar-Hillel for not foreseeing the arrival of the internet. The internet has made big data accessible and omnipresent; and modern processors have the computing power to manage it, to boot. But within that accessible knowledge, computers still aren’t quite smart enough to make the sort of unprompted connections needed to help us disambiguate words in our natural languages, using extra-textual knowledge. In other words the knowledge that a pen (as in ‘writing utensil’) cannot contain a box within its dimensions, while a pen (as in ‘enclosure’) can.
Of course, computers were built to make clear decisions based on unambiguous commands. A computer that acts in unpredictable ways is viewed to be malfunctioning. However, as we explore ways of synthesizing creative thought, randomness is becoming a compelling challenge.
In 1949, when British computer scientist Alan Turing tried to make a computer emulate human thought, he included a random number generator into his Manchester Mark 1 machine, so that it could deliver occasional wild guesses on top of stringently computed answers, claiming that “if a machine is expected to be infallible, it cannot also be intelligent.”
Controlled failure is bit of a human speciality. It is estimated that the neurons of the brain misfire 30 to 90 per cent of the time. This is a very broad scope of failure. Human thought process thus doesn’t function along tried and true algorithms, but through constant trial and error. It is reasonably estimated – as summarised by science writer Carl Zimmer – that the human brain runs on about 10 watts of energy a second. In terms of human bio power, this translates into 250–300 calories per day. Your brain runs on a Mars bar.
Meanwhile, Stanford University’s Kwabena Boahen, (who is following Turing’s path by working on Neurogrid, a hardware platform that mimics the working of the inner cortex) estimated it would take around 10 megawatts to power a processor built the current way that could mimic human level intelligence. That’s the energy output of a mid-sized power station.
Of course Turing also developed the concept of the Turing test to determine a machine’s ability to exhibit intelligent behavior equivalent to that of a human. The task to be solved? Holding a conversation with a human in a natural language. No machine has passed the test, yet.
Perhaps asking computing systems as we’ve previously built them to master the human brain’s major frame of reference is a bit like expecting a fish to climb a tree. So, instead of using the internet to continue our attempts to teach computers human language, we opted to tab it to speak computer: raw numerical data.
Statistical MT – which powers Google Translate – is based on the idea that if you feed a computer (with its massive memory but non-existent capacity for improvisation and leaps of logic) enough data in the shape of parallel texts in two languages, it will be able to spot and recreate the statistical patterns between them. The upside of this approach is that the system is self-learning, not in need of intense human curation like the closed rule-based MTs. Once the command is given, all you need to do is keep feeding the machine material so it can grow its corpus – like the Audrey II plant from The Little Shop of Horrors. (A corpus is a body of parallel texts in two or more languages. Like the Rosetta Stone, but much, much larger in volume; anywhere upwards of 30 million parallel words is considered a decent training corpus.)
The way the machine does this is by trawling parallel texts of a million or so sentences in two languages and breaking them down into small word groups of two or three, called n-grams. Once that’s done, the system can distinguish patterns between the two parallel texts, drawing the rules of translation from the order in which n-grams appear on both ends.
In so doing, the system takes all its cues from its corpus, with no grammatical guidance. It doesn’t need it. All it sees is which jumble of English letters correlates with which jumble of French letters, in relation to its nearest secondary jumble of words.
The human mind attaches layers of meaning to every sentence – the machine only recognizes strings of commands.
However, as Gestalt theory, a school of thought from visual culture, teaches: it is impossible to understand the whole by merely examining its parts. The statistical approach has issues.
James Fallows explained a central one with Google Translate feeding itself from the same bowl it spits up into – the internet – for The Atlantic: “Computerized translations are better than nothing, but at best they are pretty rough. Try it for yourself: Go to the People’s Daily Chinese-language home site; plug any story’s URL into the Google Translate site; and see how closely the result resembles real English. You will get the point of the story, barely. Moreover, since these side-by-side versions reflect the computerized-system’s current level of skill, by definition they offer no opportunity for improvement.”
That’s the problem. The more of this auto-translated material floods onto the world’s websites, the smaller the proportion of good translations the computers can learn from. In engineering terms, the signal-to-noise ratio is getting worse… This is the computer-world equivalent of sloppy overuse of antibiotics creating new strains of drug-resistant bacteria.
On a more nuanced but equally detrimental level, consider also that the problem in approaching new texts on the crutches of old ones is that old thought structures will corrupt the content. For instance, German has gendered nouns, including job titles. A male engineer would be ‘Ingenieur’, a female one ‘Ingenieurin’. The problem with corpora of pre-existing texts is that they are, well, old.
“Given that male pronouns have been over-represented throughout history in most languages and cultures, machine translation tends to reflect this historical gender bias,” says Nataly Kelly, VP at Smartling, a cloud-based enterprise translation management company. This means that statistical MT erases female engineers and male nurses as it churns.
Presently, online MT relies on analysis. But natural languages, even at official standard levels, aren’t analytical. Their words’ meanings morph in the hands of time, context and intonation, because humans aren’t consistent in their use of language, themselves.
It follows that for now, MT delivers best results with scientific and technical writing, anything that adheres more strictly to formulas. Wherever the use of language deviates from standard, where it is more colloquial or artistic, MT falters. This impacts concepts that have strong cultural roots. Researchers at UCLA raised red flags this summer to draw attention to the fact that a lot of traditional Chinese medicine is lost in translation, because many of its concepts have no English language correlation; they are tied to an understanding of the Chinese language’s culture which cannot be gained without cultural immersion.
Supposing MT is on its way to producing accurate, semantically sound text documents, we’d still need the human hand, as content translation doesn’t end with a true text document.
On the simplest level, there’s shrinkage and expansion of text – different languages take varied amounts of line space – longer or shorter or simply a different number of words – to communicate the same content. German lines run up to 30 per cent longer than English ones, which can seriously compromise a layout when re-flown automatically without attention from a designer. Not to mention that the higher density of capital letters in German impacts text colour. Then there are quotation marks, which come in a rainbow of cultural applications and shapes. And while we’re talking punctuation, consider also the inverted exclamation and question marks in written Spanish.
And this is only for translation between languages using the Latin alphabet. Ideograph languages such as Simplified Chinese are often hard to balance for uniform colour due to imbalanced symbols. In any case, they will need a much more generous leading (space between lines) to remain legible due to their higher stroke count (the amount of lines that make up a character) in a single character, much like the syllable blocks of Korean. As for reading direction, Japanese tategaki format is read from right to left, as is Arabic, which, additionally, doesn’t hyphenate, but has other ways to solve justification issues. Kashida justification elongates characters (rather than the white space between them) to compensate text colour.
In, short, text representation and its meaning changes with the language, like gestures do when they are spoken. To appropriately set a translated text, you’d need a translation engine of its own. Or, more realistically, a well-versed typographer.
In its constant drive towards blessed uniformity, Google has an ongoing project that aims to design a universal typeface family that supports every natural language. The family is called Noto, it currently covers 30 different scripts and is comprised of over 100 fonts.
Text to text translation is only the beginning. Google is working full throttle on instantaneous voice translation to be integrated into Android phones, while Samsung has acquired MT mammoth Systran to retain a cutting edge for its own products. Meanwhile, Microsoft previewed a breakthrough demo of Skype Translator in 2014, which runs real-time translation of Skype video calls in over 45 languages. YouTube should be very interested in this, as it has been working on instant subtitling options to increase accessibility for deaf users. Microsoft, again, is following suit, with impressive work by its Chinese development team who are making use of the Kinect camera to provide instantaneous MT of sign language. In 2013, the global translation market was worth an estimated $34 billion, and is predicted to grow at a rate of over five per cent per year.
MT is anything but a niche market, but for the past two decades, the focus on driving down the cost of translation by teaching it to computing systems that are ill-equipped to do the job means that we’re currently working with lackadaisical tools in a field where demand will keep growing.
To meet that demand and clean up its data, Google Translate has an improvement function that lets users enter suggestions for smoother translations. This is a very direct interaction, but there are different, incentivised ways to put human minds en masse to work on improving MT data sets.
The free language-learning app Duolingo is ambitiously described by its developers as a push towards “translating the web”. The mind behind Duolingo is Luis von Ahn, who has a previous track record of providing humans with a perk so they help machines handle human output: his reCaptcha verification system lets online users prove they’re human by deciphering a scanned text computers can’t read. Yet. Because the data they feed into the system teaches computers to do the job in the future, which greatly improves the accuracy of book digitisation.
Duolingo is a gamified learning environment to keep students engaged, especially at early stages. Once a user has progressed to higher proficiencies, they are given text chunks to translate so they can hone their skills. Duolingo then applies an algorithm to users’ cumulative translations to determine a final version to be sold commercially.
As long as computers cannot emulate a network of failing and improvising neurons jacked up on a Mars bar, we’re needed to provide it.
In terms of a five- to 10-year horizon, machine-assisted translation networks will surely prove very successful by crowdsourcing translation to user communities. One way to achieve this cooperation is classical remuneration. However, this is unlikely economically feasible on a large scale. Another is Duolingo’s approach of incentivising assistance with a smart knowledge bartering system. A third way is to rely on goodwill and enthusiasm.
Members of online fan community Viki.com band together in volunteer tag teams to produce subtitles for video content in over 200 languages – for what many would consider to be even less than a chocolate bar. After the latest episode of a Korean drama, or Mexican telenovela, or UK mini series has aired in its home market, Viki holds regional or international rights for that content and makes the video available for streaming on its website. Teams of volunteers descend on the video, segment it into manageable units, and start writing and timing subtitles. Meanwhile, fans waiting for content at the front-end receive real-time information on the percentage of the video that has been subtitled in their preferred language. The most popular shows usually have teams of 10–20 translators, who manage to have the content fully subtitled in English just a few hours after its first broadcast, with other languages trailing within the week.
The translators of Viki commit considerable amounts of their time and energy to these projects, simply for the fun of it. For recognition in the community and the pleasure of showing others content they love.
This love is a strong motivator and guarantor for quality. “Because the community is so very passionate about the content, they really care about getting the nuance of the original language and its meaning across,” says Viki Community Director Mariko Fritz-Krockow. That said, Viki does offer a coveted bonus for its well-established and most reliable community members: Qualified Contributor status – the biggest perk of which is access to all content available through the Viki site, free from regional licence restrictions. The reward of a legal all-you-can-watch.
Fans have translated video content for other fans since the first torrents went live. This means traditional licence holders trying to spread content into foreign markets have been up against a formidable online army of volunteer labourers who take joy and pride in taking down the language barriers for the rest of the world. The development of high-functioning MT systems is a lucrative goal for the culture sector as it speeds up translation to match consumer’s expectations of quick turn-overs, and Viki has managed to domesticate – and legalise – the hydra.
Viki’s Director of Communications, Michelle Laird, stresses the social nature of the site’s translation process, with no plans of going automated: “It’s so great to see communities pop up and grow. One of our most active ones is actually in Romania. And its members are absolutely amazing about harnessing and teaching each other how to translate specifically into Romanian. Since launching out of beta in 2010, we’re now at over 200 languages. A lot of Taiwanese content is getting really popular at the moment, and there is now also a lot of Chinese content that has never been distributed outside of China. Conversely, Hollywood content is becoming more popular across Asia. It’s exciting to see these developments.”
Asked about its most desirable future developments to improve the process further, Viki agreed that its focus is to continue reiterating tools to help the community communicate. By doing so, volunteers may better navigate idioms and metaphors together.
Meanwhile, Turing-level MT would eradicate the necessity to meditate on the cultural backgrounds of metaphors and figures of speech. It would make everyday interactions more serviceable, but arguably not contribute to a deeper understanding between cultures.
“Because language plays such a fundamental part in connecting each of us as thinking creatures with the world around us, the subtle nuances of language (which are different even in similar tongues, say the Latin-derived Spanish and Portuguese) actually shape how we think about the world. Learning something of how somebody else speaks from a foreign country actually helps you to understand their mindset a little,” wrote Kit Eaton on Google’s foray into simultaneous voice translation.
We’re not exactly tantalizingly close to universal MT. Back in 1954, the developers behind IBM 701 were pretty confident that translation would be fully in the circuits of computers “in the next few years” after it had chomped through those 49 sentences of scientific writing on Russian chemistry. And where are we today, over sixty years on? Google Translate currently covers just 80 of the estimated 6,000–7,000 languages spoken on the planet. Saying we’re a long way off is not fatalistic. In part because removing the human mind from translation might not actually be a utopia after all.
Philosopher Alan Wilson Watts said: “It is hard indeed to notice anything for which the languages available to us have no description.” This reflects the problem Chinese medicine faces in its dilution in translation.
Ultimately, the morsels that get lost in translation create breadcrumb trails into the backyards of people’s cultures. If those breadcrumbs vanish, we’ll be less likely to follow them down a new path.